Antimicrobial resistance (AMR) has become a significant threat to public health worldwide. *
AMR diagnostic strategies such as antibiotic susceptibility testing (AST) help provide clinicians with sufficient data to reach informed decisions and promote the administration of narrow-spectrum antibiotics4. In turn, treatment de-escalation is vital in reducing the spread of AMR while maintaining therapeutic efficacy for the patient, shortening hospital stay durations, and reducing healthcare-associated costs. *
Nanomotion technology is based on atomic force microscopy (AFM) and has been proposed as potential means of circumventing the time to result limitations of other methods by measuring bacterial viability and response to antibiotics in a growth-independent manner. *
Here, a functionalized AFM cantilever oscillates in response to bacterial vibrations. Conditions that alter bacterial vibrations, such as drug exposure, modulate AFM cantilever oscillations, and these changes can be detected, measured, and outputted using an optical read-out system. *
Nanomotion technology can distinguish the differing responses of resistant and susceptible bacteria to antibiotic treatment and has already been employed to detect antibiotic susceptibility for several different microorganisms. *
In the article “Accurate and rapid antibiotic susceptibility testing using a machine learning-assisted nanomotion technology platform” Alexander Sturm, Grzegorz Jóźwiak, Marta Pla Verge, Laura Munch, Gino Cathomen, Anthony Vocat, Amanda Luraschi-Eggemann, Clara Orlando, Katja Fromm, Eric Delarze, Michał Świątkowski, Grzegorz Wielgoszewski, Roxana M. Totu, María García-Castillo, Alexandre Delfino, Florian Tagini, Sandor Kasas, Cornelia Lass-Flörl, Ronald Gstir, Rafael Cantón, Gilbert Greub and Danuta Cichocka describe a rapid growth-independent phenotypic AST that uses a nanomotion technology platform to measure bacterial vibrations. *
Nanomotion-based AST strategies utilize technologies that are well-established in atomic-force microscopy (AFM). The nanomotion detection system described in the article is based on an AFM setup for cantilever-based optical deflection detection. *
However, in contrast to standard AFM devices, in this device the light source and the photodetector are placed below the AFM cantilever to facilitate the experimental workflow. A light beam, focused at the AFM cantilever end, originates in a superluminescent diode (SLED) module (wavelength: 650 mm, optical power: 2 mW), is reflected, and reaches a four-sectional position-sensitive photodetector that is a part of a custom-made precision preamplifier. *
The flexural deflection of the AFM cantilever is transformed into an electrical signal, which is further processed by a custom-made dedicated electronic module and recorded using a data acquisition card. The device is controlled using a dedicated AST software. *
The custom-made tipless AFM probes used for the described experiments are quartz-like tipless cantilevers with a gold coating acting as a mirror for the light beam (NANOSENSORS™ uniqprobe SD-qp-CONT-TL, typical spring constant: 0.1 N/m, AFM cantilever length × width × thickness: 130 × 40 × 0.75 µm, typical resonant frequency in air: 32 kHz from the NANOSENSORS Special Developments List). *
During an AST experiment, bacterial nanoscale movements actuate the AFM cantilever to deflect in specific frequencies and amplitudes. *
Machine learning techniques are applied to analyze a large dataset encompassing 2762 individual nanomotion recordings from 1180 spiked positive blood culture samples covering 364 Escherichia coli and Klebsiella pneumoniae isolates exposed to cephalosporins and fluoroquinolones. *
The training performances of the different classification models achieve between 90.5 and 100% accuracy. Independent testing of the AST on 223 strains, including in clinical setting, correctly predict susceptibility and resistance with accuracies between 89.5% and 98.9%. *
The study shows the potential of this nanomotion platform for future bacterial phenotype delineation. *
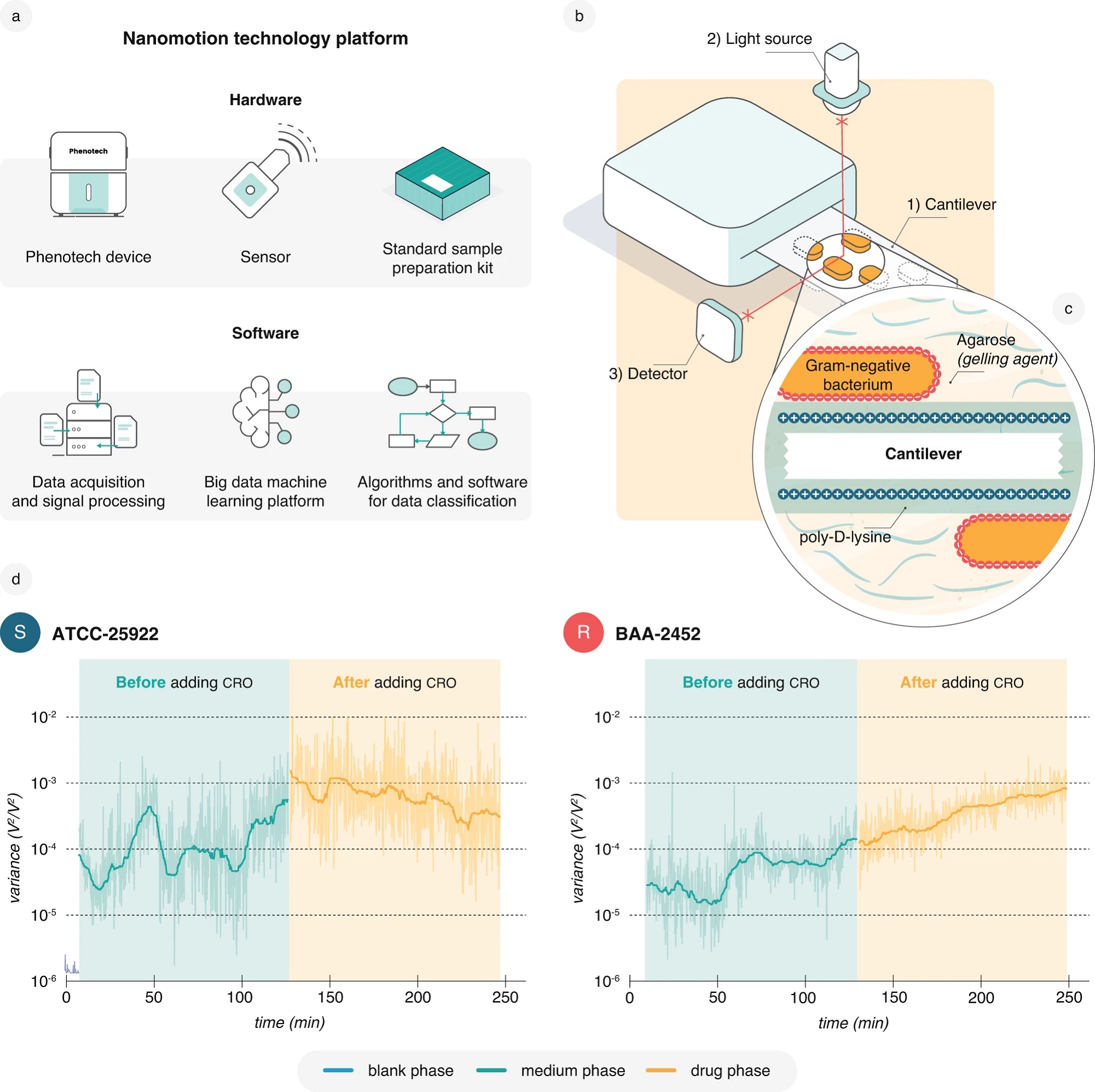
Fig. 1 from Alexander Sturm et al. “Accurate and rapid antibiotic susceptibility testing using a machine learning-assisted nanomotion technology platform”:
Nanomotion detection and recording platform.
a Representation of the components of the nanomotion technology platform. b A representation of the nanomotion measurement setup with the (1) bacteria-loaded cantilever, (2) superluminescent light emitting diode (SLED) = light source, and (3) photodetector. c Schematic illustrating Gram-negative bacteria attached to the cantilever. Prior to attachment, bacteria are dispersed in gelling agarose while the cantilever surface is functionalized using positively charged poly-D-lysine. The gelling agent proved beneficial for an even distribution and stability of the bacterial attachment. d Representative standard 4-h nanomotion recordings with a 2-h medium phase (50% LB medium) followed by a 2-h drug phase with 32 µg/ml CRO for the E. coli reference strains ATCC-25922 (S, susceptible) and BAA-2452 (R, resistant). These recordings form the basis for using nanomotion to conduct AST. This study contains 219 recordings of ATCC-25922 and 225 recordings of BAA-2452 exposed to 32 µg/ml CRO with similar results. Data are available in the source data file.
*Alexander Sturm, Grzegorz Jóźwiak, Marta Pla Verge, Laura Munch, Gino Cathomen, Anthony Vocat, Amanda Luraschi-Eggemann, Clara Orlando, Katja Fromm, Eric Delarze, Michał Świątkowski, Grzegorz Wielgoszewski, Roxana M. Totu, María García-Castillo, Alexandre Delfino, Florian Tagini, Sandor Kasas, Cornelia Lass-Flörl, Ronald Gstir, Rafael Cantón, Gilbert Greub and Danuta Cichocka
Accurate and rapid antibiotic susceptibility testing using a machine learning-assisted nanomotion technology platform
Nature Communications volume 15, Article number: 2037 (2024)
DOI: https://doi.org/10.1038/s41467-024-46213-y
Please follow this external link to read the full article: https://rdcu.be/dFEEa
Open Access: The article “Accurate and rapid antibiotic susceptibility testing using a machine learning-assisted nanomotion technology platform” by Alexander Sturm, Grzegorz Jóźwiak, Marta Pla Verge, Laura Munch, Gino Cathomen, Anthony Vocat, Amanda Luraschi-Eggemann, Clara Orlando, Katja Fromm, Eric Delarze, Michał Świątkowski, Grzegorz Wielgoszewski, Roxana M. Totu, María García-Castillo, Alexandre Delfino, Florian Tagini, Sandor Kasas, Cornelia Lass-Flörl, Ronald Gstir, Rafael Cantón, Gilbert Greub and Danuta Cichocka is licensed under a Creative Commons Attribution 4.0 International License, which permits use, sharing, adaptation, distribution and reproduction in any medium or format, as long as you give appropriate credit to the original author(s) and the source, provide a link to the Creative Commons license, and indicate if changes were made. The images or other third party material in this article are included in the article’s Creative Commons licence, unless indicated otherwise in a credit line to the material. If material is not included in the article’s Creative Commons licence and your intended use is not permitted by statutory regulation or exceeds the permitted use, you will need to obtain permission directly from the copyright holder. To view a copy of this licence, visit https://creativecommons.org/licenses/by/4.0/.